U of T course on brain-machine interfaces introduces undergrads to next-gen health care
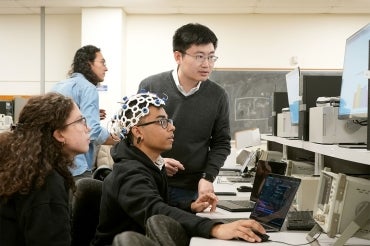
Assistant Professor Xilin Liu, standing, guides students Mona Murphy, left, and Nishant Kumar, right, as they analyze Kumar’s EEG (photo by Matthew Tierney)
Published: January 5, 2024
Undergraduate students taking a new University of Toronto course have to use their brains in more ways than one.
Called Interfacing and Modulating the Nervous System (ECE441), the course in the Edward S. Rogers Sr. department of electrical engineering and computer engineering in the Faculty of Applied Science & Engineering introduces fourth-year students to neuromodulation, a multidisciplinary area that draws on knowledge about signal processing, control theory, electronics and machine learning.
Devices employing neuromodulation deliver therapeutic stimulation to targeted areas of the brain and are used to treat a range of conditions, including chronic pain, neurological disorders such as Parkinson’s disease and epilepsy, depression, spinal cord injuries and hearing or vision loss.
During one recent lab session, students Jannis Gabler and Aurora Nowicki said they were amazed by what they could learn after they hooked up a team member.
“We were measuring electrical activity in his visual cortex,” Gabler says. “Just by extracting data from his signature, we could tell whether his eyes were closed or not.”
The course was developed through the combined efforts of faculty members Xilin Liu and Ervin Sejdić, as well as the CRANIA Neuromodulation Institute. Support from the department included the purchase of specialized equipment – biosensing headsets that allow students to acquire an electroencephalograph (EEG) – and technical support from Afshin Poraria, director of teaching labs, and lab manager Iman Makhmal Koohi.
Liu, an assistant professor in the electrical and computer engineering department, says hands-on work is a crucial component of the course, which exposes students to neural interfacing techniques and applications at a time when many are considering graduate research or starting their career.
While he notes that universities such as MIT and Stanford University offer similar courses, he says they tend to be geared toward graduate students.
“Introducing such a course at the ECE undergraduate level is quite a unique approach,” he says. “By leveraging the strengths of ECE labs, we implemented hands-on experiments enabling students to collect, analyze and modulate their own EEG signals in real-time.”
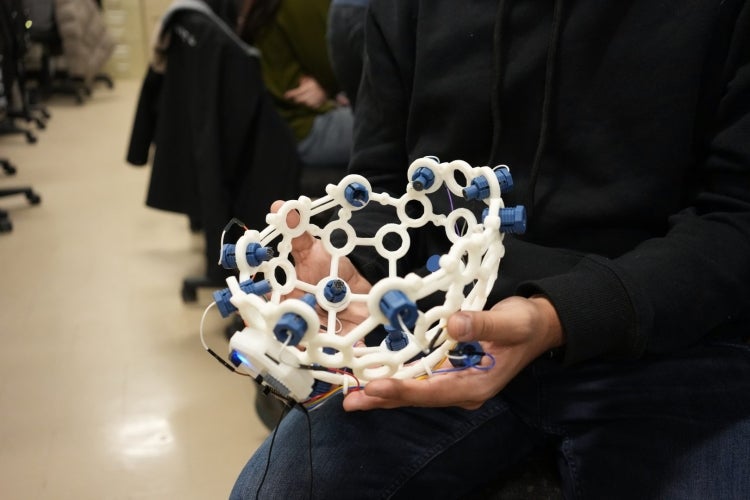
The course material combines Liu’s teachings on electronics and neural interfacing technology with Sejdić’s work on signal processing, control theory and machine learning. It also includes guest lecturers with various clinical expertise. They include Milos Popovic, a professor in the Institute of Biomedical Engineering (BME), and Taufik Valiante, a scientist at the University Health Network and an associate professor of surgery in the department of surgery in the Temerty Faculty of Medicine with a cross appointment at BME – both early supporters of the course.
During off-campus excursions to nearby hospitals and clinical settings, such as Toronto Western Hospital, the students hear from neurosurgeons and neuroscientists about real-world advancements in this field.
“The first lecture was one of the most interesting I’ve ever had at U of T,” Nowicki says.
“We learned early on how little we understand the brain. It’s incredibly complex,” adds Gabler.
Liu says we may never have an accurate general model of the brain, which means therapeutic determinations must be considered on patient-by-patient basis – a challenge for clinicians.
“You cannot keep people in the hospital for a long time just to monitor the progression of the disease,” Liu says. “And optimization done at the hospital might only be calibrated for the specific time of day, or might not work when they go back home, or while asleep.”
But wearable or implantable neural devices that use machine learning are ideally suited to address this challenge because they collect large amounts of data from patients over long periods of time. With this data, machine learning can learn the optimal timing and parameter configuration.
The field is growing so fast that the electrical and computer engineering department looks forward to expanding its course offerings in neurotechnology, says Deepa Kundur, a professor and department chair, who adds that this course helps build a strong foundation in the field.
“It’s an excellent example of how bringing awareness of cutting-edge applications into the classroom setting, through access to research labs and a diverse instructor team, allows students to see the incredible potential opportunities for their electrical and computer engineering skill set,” she says.
The department also plans to make equipment available to students outside the class. The instructors are working with the student club NeuroTECH to organize workshops and hands-on sessions.
Nowicki, for one, recommends the course to fellow students even if they’re not considering a future in health care.
“You start to look at the brain differently, the mechanisms of its disorders, what clinicians and neuroengineers are doing to treat them,” she says. “And so many people have friends or a family member who has experienced a disorder.”