U of T computer scientist takes international prize for groundbreaking work in AI
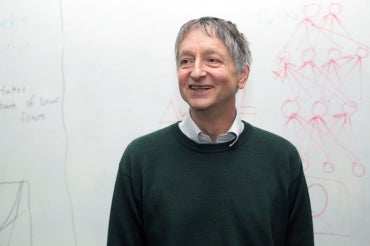
Published: January 18, 2017
Geoffrey Hinton, a University Professor in the department of computer science at the University of Toronto and VP Engineering Fellow at Google, has received the BBVA Foundation Frontiers Award in information and communications technology for his “pioneering and highly influential work” to endow machines with the ability to learn.
It marks the second year in a row that a U of T computer science researcher has won the award. Last year, University Professor Stephen Cook was honoured for his pioneering and influential work on computational complexity.
The BBVA Foundation Frontiers of Knowledge Awards were established in 2008 to recognize outstanding contributions in a range of scientific, technological and artistic areas, along with knowledge-based responses to the central challenges of our times.
The international award is the latest honour for Hinton, whose revolutionary contributions to artificial intelligence (AI) have enabled such developments as speech and image recognition systems, personal assistant apps like Siri, driverless cars, machine translation tools and language processing programs.
Read about Hinton in The New York Times
His work has also advanced the use of medical images to diagnose whether a tumor will metastasize and the search for useful molecules in new drug discovery. Virtually any field of research that requires identifying and extracting key information from massive data sets has benefitted from advances stemming from Hinton’s work.
Hinton’s approach draws on the way the human brain is thought to function
Known as deep learning, Hinton’s approach draws on the way the human brain is thought to function, with attention to two key characteristics: its ability to process information in a distributed fashion with multiple brain cells interconnected, and its ability to learn from examples.
The computational equivalent involves the construction of neural networks – a series of interconnected programs simulating the action of neurons – and, as Hinton describes it, “teaching them to learn.”
“The best learning machine we know is the human brain,” says Hinton. “And the way the brain works is that it has billions of neurons and learns by changing the strengths of connections between them.”
“So one way to make a computer learn is to get the computer to pretend to be a whole bunch of neurons, and try to find a rule for changing the connection strengths between neurons, so it will learn things in a way similar to the brain.”
The idea behind deep learning is to present the machine with lots of examples of inputs, as well as desired outputs. “Then you change the connection strengths in that artificial neural network so that when you show it an input it gets the answer right.” Hinton’s research has focused on discovering what the rules are for changing these connection strengths. He views this as the path leading to a new kind of artificial intelligence where the computer learns from its own experience.
Neural networks are far from a new thing
Although their most important applications have emerged only recently, neural networks are far from a new thing. When Hinton began working in artificial intelligence – spurred by a desire to understand the workings of the human brain that had initially taken him into experimental psychology – his colleagues were already moving away from the neural networks that he defended as the best way forward. The first results had not lived up to their promise, but Hinton chose to persevere against the advice of his professor and despite his failure to raise the necessary research funding in his home country, the United Kingdom.
His solution was to emigrate, first to the United States and subsequently Canada, where he joined U of T and was at last able to form a team and advance his work on neural networks.
By the mid-2000s, results came in that would draw scientists back to the neural network strategy. Hinton had created an algorithm capable of strengthening the connections between artificial networks enabling a computer to “learn” from its mistakes. In the resulting programs, various layers of neural networks processed information step by step. To recognize a photo, for instance, the first layer of neurons would register only black and white, the second layer would recognize a few rough features and so on until arriving at a face.
In the case of artificial neural networks, what strengthens or weakens the connections is whether the information carried is correct or incorrect, as verified against the thousands of examples the machine has been provided. By contrast, conventional approaches were based on logic, with scientists creating symbolic representations that the program would process according to pre-established rules of logic.
“I have always been convinced that the only way to get artificial intelligence to work is to do the computation in a way similar to the human brain,” says Hinton. “That is the goal I have been pursuing. We are making progress, though we still have lots to learn about how the brain actually works.”
By 2009, the programs Hinton developed with his students were beating every record in the AI area. His approach also benefitted from other advances in computation: the huge leap in calculating capacity as well as the avalanche of data that was becoming available in every domain. Indeed many are convinced that deep learning is the necessary counterpoint to the rise of big data. Today Hinton feels that time has proved him right: “Years ago I put my faith in a potential approach, and I feel fortunate because it has eventually been shown to work.”
Eventual triumph of personal assistants and driverless vehicles
Asked about the deep learning applications that have most impressed him, he talks about the latest machine translation tools, which are “much better” than those based on programs with predefined rules. He is also upbeat about the eventual triumph of personal assistants and driverless vehicles: “I think it's very clear now that we will have self-driving cars. In five to 10 years, when you go to buy a family car, it will be an autonomous model. That is my bet.”
As to the risks attached to artificial intelligence, particularly the scenario beloved of science fiction films where intelligent machines rebel against their creators, Hinton believes that “we are very far away” from this being a real threat.
What does concern him are the possible military uses of intelligent machines, like the deployment of “squadrons of killer drones” programmed to attack targets in conflict zones. “That is a present danger which we must take very seriously,” he says. “We need a Geneva Convention to regulate the use of such autonomous weapons.”
Condensed from BBVA release