U of T’s Geoffrey Hinton: AI will eventually surpass the human brain but getting jokes ... that could take time
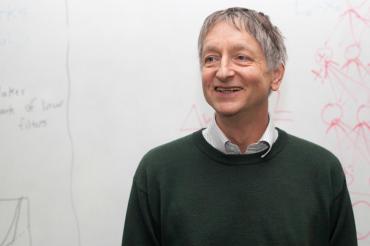
Published: December 5, 2016
U of T's Geoffrey Hinton is one of the world’s leading computer scientists, vice-president engineering fellow at Google, and the architect of an approach to artificial intelligence (AI) that will radically alter the role computers play in our lives.
Hinton, an emeritus distinguished professor in the department of computer science at the Faculty of Arts & Science, began building artificial neural networks in the 1970s. His aim was to create machines that think and learn by modelling the structure of the human brain. At the time, most researchers rejected the neural network approach to AI. But Hinton and his team kept at it.
In the past decade, their deep-learning neural networks have outstripped traditional AI in almost every benchmark. In 2013, Google acquired Hinton’s neural networks startup, DNNresearch. He was recently named to the 2016 Wired 100 list of global influencers.
His learning machines have proven immensely practical. They make self-driving cars safer, effortlessly translate between languages and will increasingly take on manual and cognitive tasks for us, at work and at home. Their ability to discover patterns in vast data sets is also helping us advance genomic medicine and develop new treatments for disease.
Read more about Geoffrey Hinton's work at Google
Hinton’s desire is simple: “I want to understand how the brain computes.”
Yet, his research has already had a huge impact on systems used by billions of people every day – and the neural network revolution has only just begun.
Hinton recently spoke with U of T News writer Jennifer Robinson about his journey in AI and what the future holds for this booming field.
What is the thing you’re most excited about right now in your research? In the Artificial Intelligence field overall?
Deep-neural networks already work very well for important tasks such as speech recognition, image interpretation and machine translation. As we get faster computers and bigger data sets, the rapid progress is sure to continue.
But I suspect the types of artificial neural network we have developed so far are not the best. There may be much better types that can learn from far less data and can give us more insight into how real brains learn. Searching for radically new types of neural network is what I am most excited about.
Why is the brain the best model to use when creating Artificial Intelligence? How soon will machines be able to compete with – and surpass – the human brain? Or has Google’s AlphaGo already proven that time is now?
Until very recently, the brain was much better than any computer at tasks like interpreting images or understanding natural language so it seemed very silly to ignore what we know about how it performs these impressive computational feats.
Recently, artificial neural networks inspired by our understanding of how the brain computes have dramatically reduced the performance gap between people and machines, and this seems to me to vindicate the idea of using the brain to provide inspiration.
I think computers will eventually surpass the abilities of the human brain, but different abilities will be surpassed at different times. It may be a very long time before computers can understand poetry or jokes or satire, as well as people.
What do artificial neural network systems actually look like? How powerful are they compared with the human brain right now? How powerful will they be in five years?
Computers can pretend to be anything that you can specify clearly.
We program them to act like simplified neurons whose output value depends on the total input they receive from other neurons or from the sensors. Each of the input lines to a neuron has an adaptive weight, and the total input is the sum of the activities on the input lines times the weights on those lines. By varying the weights, it is possible to make a neural network respond differently to the input it receives from its sensors.
The main idea of neural nets is to have a rule for how the weights on the input lines to the neurons should change as a function of experience. For example, we show a network an image and ask it to activate neurons that represent the classes of the objects that are present in the image.
To begin with, it activates the wrong neurons. But the learning rule changes the weights to reduce the discrepancy between what the network actually does and what we want it to do.
At present, it’s hard to train neural networks with more than about a billion weights. That's about the same number of adaptive weights as a cubic millimetre of mouse cortex.
In five years, we will be able to train a trillion weights which is about 1 cc of cortex. Of course, it’s possible that the learning rule we use is better than the one that the brain uses so maybe a trillion weights is all we will need to exceed the abilities of a brain that has about a thousand trillion weights.
As an AI guru at Google, you’re directing how deep learning is changing many of the products we use every day. Can you give us some recent examples of things we’re using that use AI? And what's coming next?
The brain team at Google is an extraordinary collection of highly talented engineers and scientists assembled by Jeff Dean, who designed a lot of Google's infrastructure.
When you get Google to translate for you, it now uses neural networks designed by the brain team. When you search for a document, Google uses neural nets to help it rank the results.
When you talk to the Google assistant, it uses neural nets to recognize the words you are saying. As it gets better at holding a conversation with you, it will be using more neural nets.
Hollywood and science fiction have done a great job at making us leery of possible risks in pursuing Artificial Intelligence. Do we need to be worried about the rise of the machines?
I think it will probably be quite a long time before we need to worry about the machines taking over.
A far more urgent problem is autonomous weapons such as swarms of small drones carrying explosives. These can be made now. They are as terrifying and unacceptable as biological or chemical weapons, and we urgently need international conventions to prevent their use.
Another thing we need to worry about is the use of machine learning on surveillance data to undermine political dissidents. Relying on the moral scruples of our leaders could be a mistake.
What did the funding from the Canadian Institute for Advanced Research (CIFAR) and a home at the University of Toronto mean for you – and your research?
CIFAR support made Toronto an attractive place to do research. The Natural Sciences and Engineering Research Council was also very helpful because they provided money for basic, curiosity-driven research.
These funds proved to be far more useful for revolutionizing AI than funding which was aimed at short-term industrial relevance to keep the politicians happy.
One of the most surprising things about Artificial Intelligence and deep learning is the way it’s drawing together academics from a variety of backgrounds to tackle problems together. Who are some of the most interesting – and unexpected people – you’ve worked with to date?
When I was a postdoctoral fellow in California, I used to have arguments with Francis Crick about how the brain worked. I also learned a lot from David Rumelhart who was an exceptionally insightful psychologist and deserves a lot of the credit for deep learning.
But my main collaborator back then was Terry Sejnowski, who started out in physics as a graduate student of John Wheeler (the inventor of the term "black hole") and ended up as an eminent neuroscientist.
I also did some work on tropical archaeoastronomy with an anthropologist called Edwin Hutchins who won a MacArthur (Genius Grant).
After that my main collaborators were my postdocs and graduate students, some of whom have gone on to be the directors of AI research at Facebook, Apple, and OpenAI. More recently, I have got to know a lot of brilliant scientists and engineers at Google who are too numerous to list.
As a professor at U of T for more than 27 years, how many students – ballpark – have you mentored over the years?
More than 30 graduate students have completed doctorates under my supervision, and I have also supervised quite a large number of postdoctoral fellows, master’s students and undergraduates.
If you were to draw a map of the "who’s who" in the AI/deep-learning world – most, if not all, of the big names – have a connection to you. Like Terry Sejnowski, Ilya Sutskever, Ruslan Salakhutdinov, Alex Krizhevsky, Navdeep Jaitly, Brendan Frey and so on . . . What does it feel like to have had such an impact on your field and on its future?
It feels good.
What does University of Toronto need to do next in the artificial intelligence/deep-learning field to remain a leader the field?
The University of Toronto needs to recruit a lot more faculty members in machine learning to stay at the forefront.
I am hoping that U of T will create an Institute of Machine Learning so that it can capitalize on the very large number of startups and big companies that are doing machine learning in Ontario and are desperate for more local expertise in the latest advances.
Geoffrey Hinton’s AI revolution is just one example of extraordinary innovation and impact at U of T. Learn more at www.utoronto.ca/uoft-world