U of T undergrad develops AI tool to accelerate the search for alien life
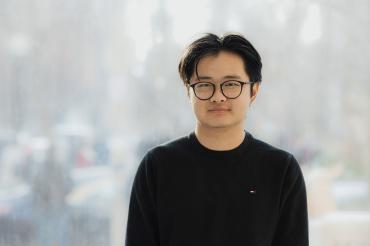
Published: January 30, 2023
Are we alone in the universe? With the help of artificial intelligence, scientists may be one step closer to finding the answer.
Led by researchers at the University of Toronto, an international team of scientists has streamlined the search for extraterrestrial life by using a new algorithm to organize the data from their telescopes into categories to distinguish between real signals and interference. Through an AI process known as machine learning, the new approach allows the researchers to quickly sort through the information and find patterns.
Since the 1960s, astronomers working on “SETI” (the Search for Extraterrestrial Intelligence) have used powerful radio telescopes to search thousands of stars and hundreds of galaxies for so-called “technosignatures,” or technologically-generated signals, on the assumption that an advanced extraterrestrial civilization would be sophisticated enough to emit such signals. Yet, despite the fact that the telescopes used for these searches are located in areas where there is minimal interference from technology like cell phones and TV stations, human disturbance still poses major challenges.
“In many of our observations, there is a lot of interference,” says Peter Ma, a U of T undergraduate student studying math and physics in the Faculty of Arts & Science who is first author on a new research paper published in Nature Astronomy that explains the technique.
“We need to distinguish the exciting radio signals in space from the uninteresting radio signals from Earth.”
By simulating signals of both types, the team has trained their machine-learning tools to differentiate between extraterrestrial-like signals and human-generated interference. They compared a range of different machine-learning algorithms, studied their precision and false-positive rates and then used that information to settle on a powerful algorithm.
This new algorithm, created by Ma, has resulted in the discovery of eight new radio signals that could potentially be transmissions from extraterrestrial intelligence. The signals came from five different stars located 30 to 90 light years from Earth. The signals were overlooked in a previous analysis of the same data, which did not use machine learning.
To the SETI team, these signals are considered notable for two reasons. “First, they are present when we look at the star and absent when we look away – as opposed to local interference, which is generally always present,” says Steve Croft, project scientist for Breakthrough Listen on the Green Bank Telescope. “Second, the signals change in frequency over time in a way that makes them appear far from the telescope.”
However, Croft notes that when you have a dataset containing millions of signals, signals can occasionally have the same two characteristics just by sheer chance. “It’s a bit like walking across a gravel path and finding a stone stuck in the tread of your shoe that seems to fit perfectly.”
For this reason, even though the eight signals appear the way the team expects extraterrestrial signals to look, the researchers are not yet convinced that they are from extraterrestrial intelligence – at least until they see the same signal again. When brief follow-up observations were done using the Green Bank Radio Telescope, the patterns that could indicate extraterrestrial signals were not found. More observations and analyses are underway.
A member of Victoria College, Ma refers to the algorithm that he created as a combination of two subtypes of machine learning – supervised learning and unsupervised learning. Called “semi-unsupervised learning,” his approach involves using supervised techniques to guide and train the algorithm in order to help it generalize, along with unsupervised learning techniques, so that new hidden patterns can be more easily discovered in the data.
Ma first came up with the idea to apply this specific algorithm to the search for extraterrestrial intelligence in a Grade 12 computer science class. Unfortunately, he says, the project confused his teachers, who weren’t sure how it could be used.
“I only told my team after the paper’s publication that this all started as a high-school project that wasn’t really appreciated by my teachers,” Ma says.
Cherry Ng, a research associate at U of T’s Dunlap Institute for Astronomy and Astrophysics and second author on the paper, says new ideas are very important in a field like SETI. “By poking the data with every technique, we might be able to discover exciting signals,” she says.
Ng, who has been working on this project with Ma since the summer of 2020, says machine learning is the way to go in an era of big data astronomy. “I am impressed by how well this approach has performed on the search for extraterrestrial intelligence,” Ng says.
“With the help of artificial intelligence, I’m optimistic that we’ll be able to better quantify the likelihood of the presence of extraterrestrial signals from other civilizations.”
Looking ahead, Ma, Ng, and the rest of the SETI team hope to expand on their new algorithm and apply it to other datasets and observatories.
Using powerful, multi-antenna radio telescopes like MeerKAT, the Square Kilometre Array, and the Next Generation VL, Ma says the team plans to scale their machine learning approach in a major way.
“With our new technique, combined with the next generation of telescopes, we hope that machine learning can take us from searching hundreds of stars to searching millions.”
The data used in this study come from the Green Bank Telescope in West Virginia, which is one of the major facilities involved in the Breakthrough Listen technosignature search project. The initiative, sponsored by the Breakthrough Prize Foundation, is the most powerful, comprehensive and intensive scientific search ever undertaken for signs of intelligent life beyond Earth.