U of T researcher launches group to help detect hidden biases in AI systems
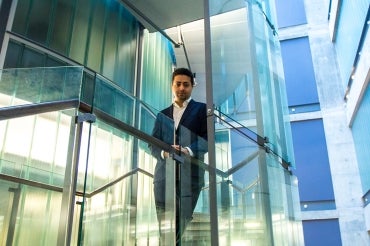
Parham Aarabi, of the department of electrical and computer engineering, helped start a research group that uncovers biases in AI systems, including some belonging to Apple, Google and Microsoft (photo by Johnny Guatto)
Published: July 5, 2021
A new initiative led by University of Toronto researcher Parham Aarabi aims to measure biases present in artificial intelligence systems as a first step toward fixing them.
AI systems often reflect biases that are present in the datasets – or, sometimes, the AI’s modelling can introduce new biases.
“Every AI system has some kind of a bias,” says Aarabi, an associate professor of communications/computer engineering in the Edward S. Rogers Sr. department of electrical and computer engineering in the Faculty of Applied Science & Engineering. “I say that as someone who has worked on AI systems and algorithms for over 20 years.”
Aarabi is among the academic and industry experts in the University of Toronto’s HALT AI group, which tests other organizations’ AI systems using diverse input sets. HALT AI creates a diversity report – including a diversity chart for key metrics – that shows weaknesses and suggests improvements.
“We found that most AI teams do not perform actual quantitative validation of their system,” Aarabi says. “We are able to say, for example, ‘Look, your app works 80 per cent successfully on native English speakers, but only 40 per cent for people whose first language is not English.’”
HALT was launched in May as a free service. The group has conducted studies on a number of popular AI systems, including some belonging to Apple, Google and Microsoft. HALT's statistical reports provide feedback across a variety of diversity dimensions, such gender, age and race.
“In our own testing we found that Microsoft’s age-estimation AI does not perform well for certain age groups,” says Aarabi. “So too with Apple and Google’s voice-to-text systems: If you have a certain dialect, an accent, they can work poorly. But you do not know which dialect until you test. Similar apps fail in different ways – which is interesting, and likely indicative of the type and limitation of the training data that was used for each app.”
HALT started early this year when AI researchers within and outside the electrical and computer engineering department began sharing their concerns about bias in AI systems. By May, the group brought aboard external experts in diversity from the private and academic sectors.
“To truly understand and measure bias, it can’t just be a few people from U of T,” Aarabi says. “HALT is a broad group of individuals, including the heads of diversity at Fortune 500 companies as well as AI diversity experts at other academic institutions such as University College London and Stanford University.”
As AI systems are deployed in an ever-expanding range of applications, bias in AI becomes an even more critical issue. While AI system performance remains a priority, a growing number of developers are also inspecting their work for inherent biases.
“The majority of the time, there is a training set problem,” Aarabi says. “The developers simply don’t have enough training data across all representative demographic groups.”
If diverse training data doesn’t improve the AI’s performance, then the model itself may be flawed and require reprogramming.
Deepa Kundur, a professor and the chair of the department of electrical and computer engineering, says HALT AI is helping to create fairer AI systems.
“Our push for diversity starts at home, in our department, but also extends to the electrical and computer engineering community at large – including the tools that researchers innovate for society,” she says. “HALT AI is helping to ensure a way forward for equitable and fair AI.”
“Right now is the right time for researchers and practitioners to be thinking about this,” Aarabi adds. “They need to move from high-level abstractions and be definitive about how bias reveals itself. I think we can shed some light on that.”