U of T computer science researchers tackle voter distribution and how it affects gerrymandering in the U.S.
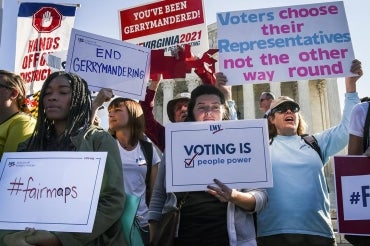
Published: July 16, 2018
In the early 19th century, Massachusetts Governor Elbridge Gerry reconfigured the state districts to guarantee the U.S. Senate election of Democratic-Republican candidates in opposition to the Federalists. It was said the new boundary of Essex County looked like a salamander, but an opponent quipped it looked more like a gerrymander.
Gerrymandering, or manipulating, electoral boundaries continues in the U.S. today, and is a hotly debated political issue. HBO’s Last Week Tonight host John Oliver recently warned that gerrymandering is allowing elected legislators to draw their own district lines and pick their own voters.
To gerrymander is, however, computationally complex.
“There is a well-known phenomenon that the Democrats are packing themselves in cities and the Republicans are staying in the more rural areas,” says Nisarg Shah, an assistant professor in the University of Toronto's department of computer science. “As voters move around, we wanted to analyze what would be the effect of this process on the ability each party has to gerrymander, and so we first needed to define what it means to have a certain amount of gerrymandering power.”
U of T theoretical computer scientists will present their early findings this week, at the International Joint Conference on Artificial Intelligence (IJCAI) in Stockholm, Sweden. IJCAI, the premier international gathering of researchers in AI, takes a broad view of the field, including problems of automation, optimization and game theory.
In their paper, "Big City vs. the Great Outdoors: Voter Distribution and How it Affects Gerrymandering," University Professor Allan Borodin, former U of T post-doctoral researcher, Omer Lev, Shah and Tyrone Strangway, a PhD student of computer science, propose a simple model for measuring gerrymandering power.
In their simplified simulation, Shah says they created 32 districts on a grid. If a party has only 60 per cent of the votes, but by dividing the districts in a certain way they can ensure their party can win 90 per cent of the districts, then their gerrymandering power is 30 per cent.
“If you imagine Democrats packing themselves into these small, dense regions and the Republicans staying around in the rural regions, then it limits the gerrymandering power that either party has,” says Shah.
“This is because you have this nice separation between the borders of the two parties, so the only flexibility you have to gerrymander is at the edge of the somewhat suburban areas, where there are some Democrats and some Republicans mixed in.”
The researchers say as a party moves around in this fashion, a party’s gerrymandering power may increase a little, and but then it goes down, or levels off.
“One quantity we need to be able to compute is: What’s the maximum percentage of seats a party can win? And that's the computationally hard question,” says Shah.
University Professor Allan Borodin and Assistant Professor Nisarg Shah, both of U of T's department of computer science
To find the highest number of seats, Shah says an optimal algorithm didn’t scale, but rather timed out without a result. Instead, they have a method that estimates this quantity. Their next steps would be to refine methods, and apply to 2016 election data.
“That's the way you start in theory,” says Borodin of their current model. “We don't have good ways of defining what the geometric conditions are for what a district has to look like, other than that has to be connected.”
The researchers argue that this a difficult problem, computationally. Shah says it’s an area where designing better algorithms that can gerrymander can actually be a good thing, to precisely measure how much power a party has to change the course of an election.
They point to North Carolina, Pennsylvania, Wisconsin and Texas as extreme in their gerrymandering tactics. The Voters Rights Act makes it illegal to gerrymander to disadvantage a racial minority, but Republican representatives in North Carolina have openly said they’ve redrawn districts for partisan reasons. The only reason the Republicans won 10 out of 13 districts, the researchers say, was because they could not calculate how to win 11.
“To compute their gerrymandering power, as computer scientists, we would need to be sure that there is no way to win 11. That's the challenge – to find the provably optimal answer,” says Shah.
However, not all gerrymandered districts are bad. The “weird” shapes defining these boundaries might signal geographic limitations, such as a lake, or help bring communities of interest together, say the researchers, rather than fragmenting the population across many districts, where they’re not able to elect a candidate who represents their interests.
“The U.S. Supreme Court has to rule on this, but wants some clear-cut legalistic definition [of gerrymandering],” says Borodin. “It really requires a precise definition. But what's the right definition? You can't make it too stringent. So it leads to very interesting political questions, but also very interesting computational ones.”
Borodin says this problem is just one of many in the growing sphere of the social, political and economic sciences where computer science is now involved, and is influencing the way people think about computation. He says social choice is a growing area of interest to current researchers in the department.
“There are other questions that are related to this, about fairness and how you define fairness,” says Borodin.
This research was supported by the Natural Sciences and Engineering Research Council of Canada (NSERC).