Researchers at U of T use physics model to study spread of COVID-19 respiratory droplet ‘clouds’
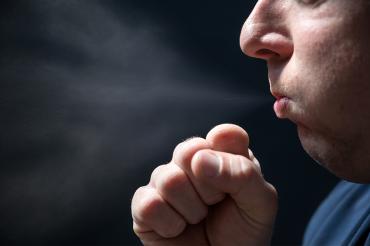
Published: December 8, 2020
After nearly a year of studying COVID-19, scientists are still grappling with fundamental questions – including understanding the dominant modes of transmission and predicting how “superspreading” events arise. A newly improved model produced by engineers and physicists could help.
Associate Professor Swetaprovo Chaudhuri of the University of Toronto Institute for Aerospace Studies and his colleagues have developed what they called a “first-principles modelling approach” to understanding the factors that affect COVID-19 spread.
Unlike epidemiological models that predict the spread of a disease based on empirical data from past outbreaks or by estimating person-to-person contact, the team’s model makes use of fundamental flow physics concepts, droplet and jet aerodynamics, evaporation and related thermodynamics. The idea was to simulate the physical path of droplets expelled by infected people along with their physical state. The model can be used to predict the probability of transmitting the infection from one person to another
While the team – which included Assistant Professor Abhishek Saha at the University of California, San Diego and Professor Saptarshi Basu of the Indian Institute of Science – outlined their findings in a paper published back in June, Chaudhuri says a new paper published in the journal Physics of Fluids takes into account that a respiratory event produces not one droplet but a jet: a cloud with a large range of droplet numbers and sizes. When these droplets evaporate, they leave behind solid nuclei, which also form part of the cloud.
“In the previous paper, we analysed lifetime and travel distance of individual respiratory droplets of different sizes,” Chaudhuri says.
The new model draws inspiration from the experimental works of Professor Lydia Bourouiba at MIT, whose team has published extensively on the fluid dynamics of disease transmission. The team also accounted for interactions between people and the cloud in a social setting, which was modelled using equations originally designed to describe collisions between molecules in a chemical reaction.
Distinctions can be made between different types of respiratory events such as coughing, breathing, singing, sneezing or even talking. The model also incorporates the most recent information about typical SARS-CoV-2 viral load and virus lifetimes.
Combining the “physics of the cloud” with the “physics of the crowd” enables the researchers’ model to produce estimates of the basic reproduction number, known as R0, under various scenarios, as well as potentially explaining how superspreading events can occur.
R0 can be thought of as the expected number of new cases that each current case will give rise to over its lifetime. If it is above one, the disease will continue to spread exponentially. If not, the pandemic will eventually burn out.
As before, the team tested their basic droplet model with an acoustic levitator, a device that uses sound waves to cause droplets of saline solution to float suspended in air. This time, the team used real saliva of “a healthy subject who was Covid-19 negative.” The experiment was performed at the Indian Institute of Science in Bangalore and results will be published in a future paper.
Inferences of the model include:
- The most lethal droplets from a cough were those with initial sizes of 10 to 50 microns. This is a size blocked by most masks. But Chaudhuri points out that, by the time they are inhaled, the droplets could shrink to less than 20 per cent of their original size.
- Droplets – and especially the dried-out nuclei that contain the virus particles – can easily spread 12 feet or further. However, with distance, the droplet cloud expands and gets diluted, which reduces the probability of infection.
- Without ventilation, droplet nuclei are persistent, meaning they remain suspended in air for long periods of time. Because of this persistence, they have the potential to infect larger numbers of people than the initial droplets.
As for advice to reduce the spread, Chaudhuri cautions that he’s not an expert on disease transmission, but says the following is consistent with the team’s model: wear a mask (three layered masks are most effective), avoid spending time indoors in public places, improve ventilation, keep as far apart as possible and also wash hands frequently.
Chaudhuri acknowledges that this model is based on an idealized scenario and includes a number of assumptions. One of the most important relates to what degree the virus particles embedded inside the droplets remain infectious after the liquid surrounding them has evaporated, a question he says is “still an ongoing research problem for virologists.”
But he says the value of a physics-based approach is that the same basic framework can be adapted to different scenarios simply by adjusting certain parameters or the boundary conditions of the analysis.
“Additional complexities in terms of room size, partitions, ventilation, could be introduced and accounted for,” he says.
The team hopes to collaborate with more researchers outside their discipline to address some of the areas where their model could be further improved. Chaudhuri stresses the multidisciplinary nature of the challenges posed by COVID-19.
“This is a problem that involves doctors, public health researchers, epidemiologists, virologists, mathematicians, computer scientists, as well as experts in aerosols and fluid mechanics,” he says. “We have to work together to gain a complete picture.”