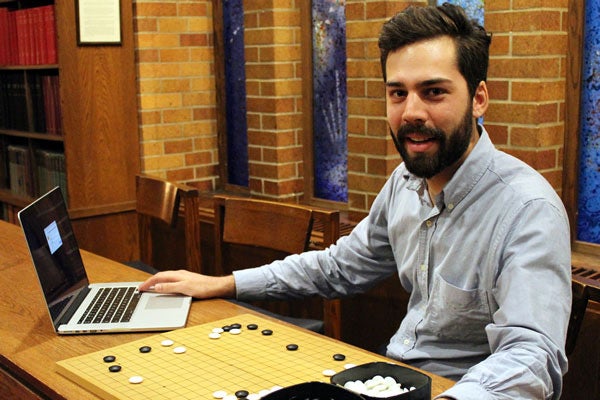
Google DeepMind's AlphaGo: meet the U of T computer scientists who helped it win
Published: February 2, 2016
Go is one of the world’s oldest games, originating some 2,500 years ago in China.
Now the latest in artificial intelligence – Google DeepMind’s AlphaGo – has set a milestone in the game once believed impossible for a computer to learn, beating European champion Fan Hui 5-0.
“The rules of Go are very simple,” says computer science PhD student and Massey College fellow, Chris Maddison. “But it’s in that simplicity that complexity arises.”
Maddison is third author of new research published in Nature and announced by Google Research on Jan. 27, detailing AlphaGo’s success at mastering the game and beating previous state-of-the-art Go-bots in 99.8 per cent of games with even stones.
Read The Globe and Mail story
Go is fundamentally a game of conquering territories. The game proceeds with two players, alternating the placement of either black or white stones on a 19 by 19 grid. The goal is to define territory using the stones, and whoever controls most of the board by the end of the game, wins.
“You might think the right thing to do is to make big blobs to cover the board,” says Maddison. “But you can capture territory by completely surrounding your opponent’s connected stones. So, professional play leads to beautiful stringy patterns that cut and connect across the board.”
Artificial intelligence research has a long history of using games as microcosmic testing-grounds. Games are very precisely defined and allow researchers to evaluate their success. Last year, Google DeepMind taught a machine to play and win at all 49 classic Atari computer games. Go has always been seen as the last classical game where humans consistently outperform algorithms.
Read more about artificial intelligence research at U of T
Part of the reason Go is so difficult, is the sheer number of possible results. Every Go game is rare and extremely unlikely to repeat. AlphaGo beat Fan Hui on games it had never seen before.
“The obvious thing to do would be to search all possible outcomes, but that’s not going to work in games like chess, and especially Go,” says Maddison.
What distinguishes AlphaGo from previous Go-bot approaches are the use of neural networks, computationally layered, banks of knowledge – and an area of research advanced by his PhD supervisor, University Professor Emeritus Geoffrey Hinton, who is also a distinguished researcher at Google.
Read more about Geoffrey Hinton
“What neural networks allow us to do, is to narrow the number of outcomes we’re going to investigate. But what they’re also very good at, is generalizing to states they’ve never seen before. So these networks learn principles and tactics. They don’t just memorize – they comprehend.”
AlphaGo on its own isn’t going to do much else. But like other neural networks that have proven to be successful in image and speech recognition, this latest test proves further confidence in these systems, which could lead to use in other applications, from disease prevention to smartphone technology.
“It’s like showing motors can move very big, heavy things – now you can apply it to other big, heavy things.”
Among the contributing authors are University of Toronto cognitive science graduate Timothy Lillicrap, a member of the Google DeepMind team, and computer science graduate alumnus Ilya Sutskever, now director of research at OpenAI, a $1-billion non-profit dedicated to artificial intelligence research.
Maddison, who took leave last year to intern at Google DeepMind based in London, England, says it’s thrilling to be part of a team that grew from a small-scale project two years ago, to the industrial-scale project of today with many researchers and engineers. And the result is most satisfying.
AlphaGo will play again this March, when it challenges Lee Sedol, the strongest and most titled Go player of the last decade.
“I’m not a particularly strong player of Go,” admits Maddison. “I think it speaks to the technology we used. I didn’t need to be. But it sparked an interest I’d like to study more.”
(Nina Haikara is a writer with the department of computer science in the Faculty of Arts & Science at the University of Toronto)